Faculty Spotlight: Qing Mai
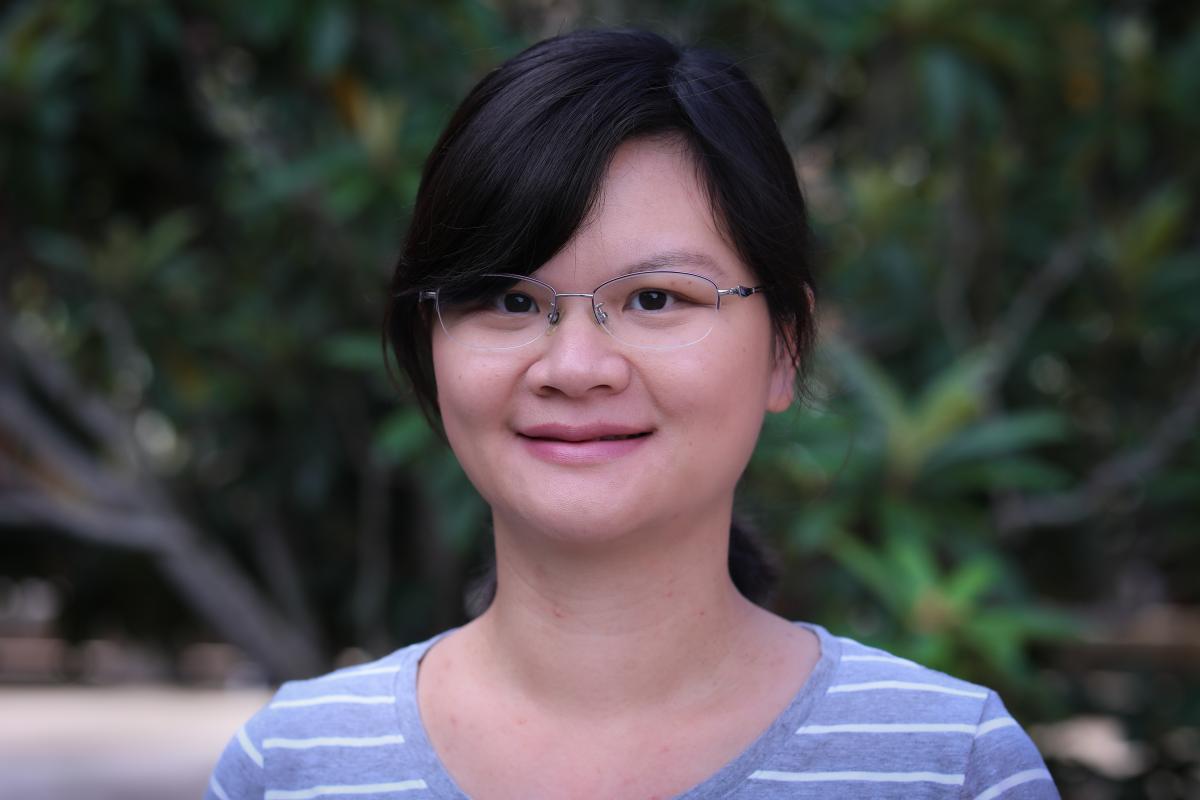
Qing Mai is a professor in the Department of Statistics, part of Florida State University’s College of Arts and Sciences. Mai earned her bachelor’s degree in statistics from Nankai University in Tianjin, China, in 2008 and her doctorate from the University of Minnesota in 2013. Her research interests lie in statistical learning, and she teaches courses on statistical inference and the study of high-dimensional data.
Tell us a little about your background, where you’re from and what brought you to FSU.
I was born in China. As a kid I had many plans, but in middle school I decided mathematics was the most fascinating subject. Even before starting my doctoral program, I was determined to become a professor and researcher so I could work on interesting problems. I interviewed at FSU for a position in the Department of Statistics and was impressed by the department’s diverse research interests. Everyone was open-minded to one another’s research and passionate about their own; I was drawn to this and joined FSU’s faculty in 2013.
Tell us about your areas of research and what inspired your interest in these topics.
I work in statistical learning, which aims to develop methods enabling computers to make accurate decisions without human supervision. I am especially interested in high-dimensional data (data in which the number of variables observed are close to or larger than the number of observations) and tensor (multi-dimensional) data. These interests are inspired by problems outside of statistics. For example, in biology, most genomic studies generate high-dimensional data because humans have so many genes. One important application of my research is assisting doctors who diagnose disease. In another example for neurologists or psychologists, measurements of adjacent brain regions can be viewed as tensor data, meaning numbers are arranged in a certain informative way.
As a statistician, I emphasize statistical perspectives to understand implications behind statistical assumptions and why certain perspectives are more successful than others by identifying their theoretical properties. For the convenience of other scientists and medical professionals who might use my methods, algorithms are developed for fast implementations across diverse fields.
Your research covers several areas within statistics. What are your most recent projects?
Recently, I have been working on unsupervised learning for modern datasets — use of machine learning algorithms to analyze and cluster unlabeled datasets. Unsupervised learning intends to uncover heterogeneity, or variability in data, an integral part of working with large datasets. When a dataset contains measurements of a large number of subjects, there are usually multiple explanations behind the data. For example, if we study many patients with the same disease, we will likely find subtypes of the disease with different causes. Unsupervised learning is especially challenging for high-dimensional datasets and tensor datasets due to large volumes and special data structures. As it is difficult to analyze these with existing methods, I am developing new methods to address challenges.
What do you want the public to know about your research? Why are your topics important?
My research is strongly motivated by real-world problems. I hope to make my methods readily available to experts in other areas if they choose to use them for their own data analysis. Most of my methods have been extensively tested on publicly available datasets and are implemented in software with a user-friendly interface for ease of use by such experts.
You are a panelist for the National Science Foundation and serve in editorial roles for several journals. Tell us a bit about how these activities contribute to your research and work at FSU.
As a panelist, I help program officers identify the most promising proposals for funding by the National Science Foundation. I read proposals from researchers across the U.S., judging their novelty and merits. As an associate editor of the academic journals Biometrics and Computational Statistics and Data Analysis, I screen submitted papers to evaluate their chance for acceptance. If there is a chance for acceptance, I then invite referees to perform thorough reviews. Based on those reviews and my own opinion, I submit recommendations to the editor.
These service roles expose me to the newest research, which greatly benefits my own work. I have the opportunity to learn what problems other researchers are interested in and what new techniques are being developed. When junior faculty members ask me for career advice, I can speak from my own experiences and what I have learned in these roles.
Who are the people or mentors who have influenced you most in your career?
I’m very fortunate to have met many insightful people, but my adviser, Hui Zou, the Dr. Lynn Y.S. Lin Professor at the School of Statistics in the University of Minnesota, influenced me most. When I started my doctoral research, he introduced me to high-dimensional statistics as it was becoming more prominent in the field, and I have witnessed its rapid expansion since then.
What is your best memory from all your time you’ve spent at FSU?
Seeing my students graduate and start their new endeavors; it is amazing to realize how much they grow in just a few years.
What is your favorite part of your job? What is most challenging?
That I can choose my own problems to study, and it is always thrilling to find a solution. The most challenging part is time management because there are so many intriguing things to do.
What are some specific goals or projects you are working towards in the coming year?
Statistical methods have underlying mathematical assumptions that justify their applications. Among these assumptions is that data should be somewhat “normal,” meaning most observations behave similarly, however, this assumption is often too restrictive for real datasets. I am working on a new framework for data analysis that automatically adapts to non-normality of data.
If your students only learned one thing from you (and of course, hopefully they learn much more than that), what would you hope it to be?
Things are rarely as difficult as we imagine, and problems are rarely as difficult as we fear. Most of the time, we can just try to do our best, and it will eventually all work out.